Oncology Company Announces Promising Ovarian Cancer Study Results
Predictive Oncology Announces Promising Results from Ovarian Cancer Study with UPMC Magee-Womens Hospital.
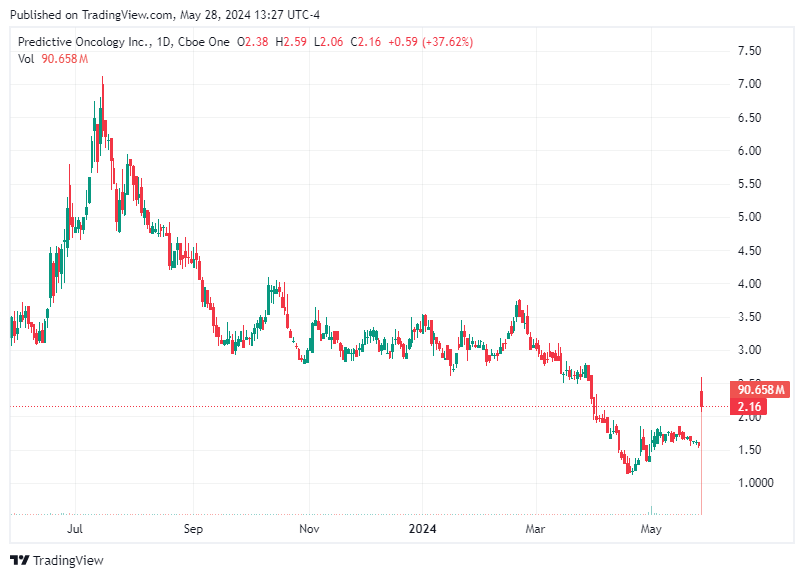
Disclaimer: The following article discusses the results of a scientific study. The information provided herein is for informational purposes only and should not be construed as medical advice. Always consult with a healthcare professional before making any decisions about your health or treatment options.
Predictive Oncology Inc. (NASDAQ: POAI), a company in AI-driven drug discovery and biologics, has announced significant positive results from a retrospective study conducted in collaboration with UPMC Magee-Womens Hospital. These results are set to be presented at the 2024 American Society of Clinical Oncology (ASCO) Annual Meeting, taking place from May 31 to June 4, 2024, in Chicago, IL. The presentation will provide insights into the use of machine learning (ML) models to predict survival outcomes for ovarian cancer patients, potentially transforming clinical management and treatment personalization.
The Study's Purpose and Scope
The primary aim of the study was to determine the efficacy of Predictive Oncology's artificial intelligence (AI) capabilities in developing ML models that could accurately predict short-term (two-year) and long-term (five-year) survival outcomes among ovarian cancer patients. This study holds profound implications for improving prognostic accuracy and tailoring treatment plans to individual patients' needs.
Dr. Robert Edwards, Professor and Chair of the Department of Obstetrics, Gynecology & Reproductive Sciences at Magee-Womens Hospital of UPMC, Co-Director of Gynecologic Oncology Research, highlights the critical need for such advancements. "High-grade serous ovarian cancer is a notoriously challenging cancer to treat, due in large part to the lack of symptoms in the early stages of disease," he stated. "While surgery and frontline chemotherapy are effective in the near-term, nearly 80% of patients will relapse in one to two years, and only 20% will be long-term survivors. The ability to employ ML to better predict patient prognoses may help with clinical management and monitoring and could serve as a decision support tool to better tailor treatment plans to individual patients."
The study's results strongly support the continued development and incorporation of ML models into daily clinical practice. Dr. Brian Orr, MD, lead investigator of the study, along with other collaborators at Magee-Womens Hospital, showcased the potential of AI and ML to transform oncology by enhancing prognostic accuracy and informing clinical decisions in real-time. Arlette Uihlein, MD, Senior Vice President of Translational Medicine and Drug Discovery and Medical Director at Predictive Oncology, emphasized the broader implications of these findings. "We believe these results highlight the potential of AI and machine learning to not only accelerate early oncology drug discovery but to assist with the clinical management of cancer patients in real-time, thereby improving survival outcomes. We also see an opportunity to leverage these findings to discover unique biomarkers that can be used by us or a partner to develop novel cancer therapeutics."
Advantages of Predictive Oncology's Unique Assets
Predictive Oncology differentiates itself from peers through several unique assets and capabilities:
Biobank: Housing over 150,000 tumor samples.
Pathology Slides: Access to 200,000 pathology slides.
CLIA-Certified Wet Lab: Equipped for advanced research and testing.
Longitudinal Patient Data: Decades of patient data that provide deep insights into cancer progression and treatment outcomes.
These resources enable Predictive Oncology to be at the forefront of leveraging AI and ML in cancer research, offering unprecedented opportunities for breakthroughs in understanding and treating ovarian cancer. The details of the presentation at the ASCO Annual Meeting are as follows:
Title: Using Artificial Intelligence-Powered Evidence-Based Molecular Decision-Making for Improved Outcomes in Ovarian Cancer
Abstract #: 448976
Session: Gynecologic Cancer
Date/Time: Monday, June 3rd, 9:00am-12:00pm CDT (10:00am-1:00pm EDT)
Presenter: Dr. Brian Christopher Orr, MD, MS, Gynecologic Oncologist at the Hollings Cancer Center, Assistant Professor, Medical University of South Carolina.
The successful execution and promising results of this study are poised to influence both future research and clinical practice significantly. The integration of AI and ML in oncology can help not only in predicting patient prognosis but also in identifying novel therapeutic targets, thereby contributing to the overall improvement of cancer treatment and patient care. Dr. Edwards and Dr. Uihlein's comments underscore the potential impact of these innovations. As Dr. Edwards noted, the ability to accurately predict patient outcomes using ML models presents an invaluable tool for clinicians, potentially leading to more personalized and effective treatment strategies. Meanwhile, Dr. Uihlein highlighted how these findings could accelerate early drug discovery and facilitate real-time clinical management, ultimately aiming to improve survival rates for ovarian cancer patients.
The collaboration between Predictive Oncology and UPMC Magee-Womens Hospital marks a significant milestone in the application of AI and ML in cancer research and treatment. The positive results of the study underscore the potential for these technologies to revolutionize oncology, providing new avenues for personalized treatment and improved patient outcomes. The upcoming presentation at the ASCO Annual Meeting will further elucidate these findings, offering the medical community valuable insights into the future of cancer treatment.
Summary of Study Methodology
The study analyzed clinical data and tumor specimens collected from 2010 to 2016. Patient data encompassed whole exome sequencing (WES), whole transcriptome sequencing (WTS), drug response profiles, and digital pathology profiles. These diverse datasets were utilized as input feature sets for training 160 multi-omic ML models. Hypothesis-free training methods were employed to classify patient survival at two- and five-year intervals. Model performance was estimated using the area under the receiver operating characteristic curve (AUROC) metric, with scores greater than 0.5 indicating higher prediction potential. Of the 160 ML models built, seven achieved high prediction accuracy at the two-year threshold, while 13 models demonstrated high accuracy at the five-year threshold. Models utilizing multi-omic feature sets outperformed those based on clinical data alone. Moreover, the top-performing models, which incorporated multi-omic data, predicted survival outcomes more accurately than any feature set used in isolation.
The integration of multi-omic data (including genetic and transcriptomic profiles) significantly enhanced the predictive capabilities of the ML models. This underscores the benefit of utilizing a comprehensive set of biological data rather than relying solely on clinical indicators. The study found that different sets of features drove the predictive accuracy for short-term versus long-term survival outcomes, suggesting varied underlying mechanisms which could be further explored in future research.
The study concluded that utilizing multi-omic ML models led to superior prediction of both short- and long-term survival in ovarian cancer patients, compared to using clinical data alone. These findings highlight the potential development of a clinical decision support tool that could revolutionize personalized treatment planning and patient management in oncology.
Dr. Edwards and Dr. Uihlein's comments underscore the potential impact of these innovations. As Dr. Edwards noted, the ability to accurately predict patient outcomes using ML models presents an invaluable tool for clinicians, potentially leading to more personalized and effective treatment strategies. Meanwhile, Dr. Uihlein highlighted how these findings could accelerate early drug discovery and facilitate real-time clinical management, ultimately aiming to improve survival rates for ovarian cancer patients.
About Predictive Oncology
Predictive Oncology is at the forefront of the rapidly expanding use of artificial intelligence and machine learning to expedite early drug discovery and enable drug development for the benefit of cancer patients worldwide. The company’s scientifically validated AI platform, PEDAL, predicts with 92% accuracy whether a tumor sample will respond to a specific drug compound, allowing for more informed selection of drug/tumor type combinations for subsequent in-vitro testing. With its vast biobank of more than 150,000 assay-capable heterogeneous human tumor samples, Predictive Oncology offers one of the industry’s broadest AI-based drug discovery solutions. This is further complemented by its wholly owned CLIA lab and GMP facilities, positioning the company as a leader in AI-driven oncological research.
Headquartered in Pittsburgh, PA, Predictive Oncology collaborates with academic and industry partners to advance the understanding and treatment of various cancers, reinforcing its commitment to improving patient outcomes through innovative technological solutions.
The collaboration between Predictive Oncology and UPMC Magee-Womens Hospital marks a significant milestone in the application of AI and ML in cancer research and treatment. The positive results of the study underscore the potential for these technologies to revolutionize oncology, providing new avenues for personalized treatment and improved patient outcomes. The upcoming presentation at the ASCO Annual Meeting will further elucidate these findings, offering the medical community valuable insights into the future of cancer treatment.
Disclaimer: The preceding article is based on the results of a scientific study. The information provided should not replace professional medical advice or consultations with healthcare professionals. Always consult with a healthcare professional regarding any health decisions or treatment plans.
As an investor it's important to stay updated with major news. Get real-time stock market alerts, news, and research by creating an account here.