Google Develops Table Tennis Robot
Google DeepMind Develops a 'Solidly Amateur' Table Tennis Robot.
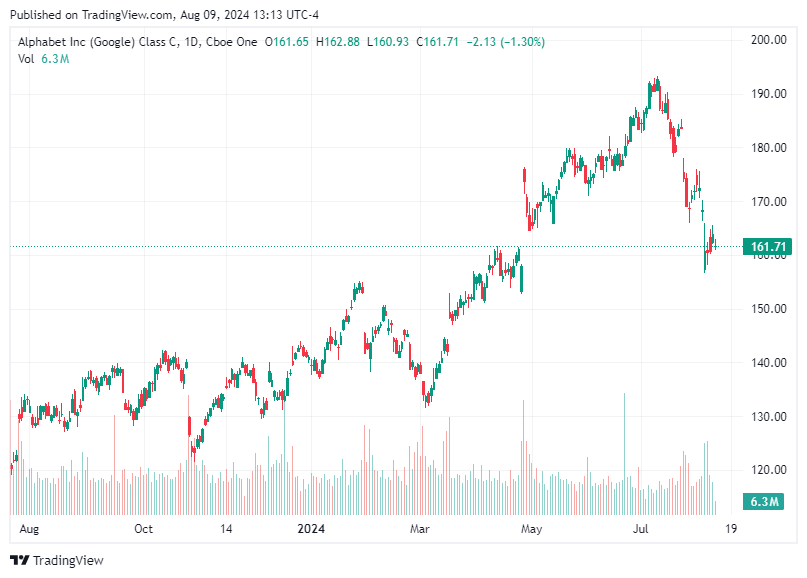
Disclaimer: The information presented in this article is based on research and data available as of the publication date. It is intended to provide a comprehensive overview of Google DeepMind's table tennis robot and is not an endorsement or promotion of any products or services.
Real-time information is available daily at https://stockregion.net
Table tennis, with its dynamic gameplay and rapid exchanges, offers a unique challenge for robotics. Google DeepMind, a leader in artificial intelligence research, has developed a table tennis robot that performs at a "solidly amateur" human level. The robot has shown proficiency against beginner and intermediate players but struggles against advanced opponents.
Historical Context: Robotics in Sports
Robotics has long found a testing ground in sports, where the combination of physical dexterity, speed, and strategic thinking provides a rich field for research and development. One of the most well-known examples is the annual RoboCup soccer competition, which began in the mid-1990s and showcases robots playing soccer. However, the use of table tennis as a benchmark for robotic arms dates back to the 1980s. The sport demands not only physical agility but also quick decision-making and strategic planning, making it an ideal testbed for robotic advancements.
The DeepMind Robotics team detailed their work in a paper titled "Achieving Human Level Competitive Robot Table Tennis." The focus of this research was to develop a robot capable of playing table tennis at a human level. The robot was designed to handle the complexities of the game, such as returning the ball, strategizing, and long-term planning. During testing, the robot demonstrated impressive results against beginner-level players, winning all matches. Against intermediate players, the robot had a win rate of 55%. However, when facing advanced players, the robot consistently lost. Overall, the system won 45% of the 29 games it played. These results indicate that while the robot has achieved a level of proficiency, there is still room for improvement.
Technical Challenges
One of the primary technical challenges highlighted by the DeepMind team is the robot's ability to react to fast balls. System latency, mandatory resets between shots, and a lack of useful data are cited as key reasons for this limitation. To address these issues, the researchers propose investigating advanced control algorithms and hardware optimizations. Potential solutions include exploring predictive models to anticipate ball trajectories and implementing faster communication protocols between the robot's sensors and actuators.
Other areas for improvement include handling high and low balls, executing backhand shots, and reading the spin on incoming balls. These challenges showcases the complexity of achieving human-level performance in a sport that requires split-second decisions and precise movements. While the immediate application of a table tennis-playing robot may seem limited, the research conducted by DeepMind has broader implications for the field of robotics. The development of a robot capable of playing a sport at a human level represents a level up towards achieving human-level performance in various real-world tasks. The insights gained from this research could inform the development of generalist robots capable of performing multiple useful tasks and interacting safely with humans.
DeepMind's work on policy architecture, the use of simulation to operate in real games, and the ability to adapt strategies in real-time are all critical components that could be applied to other areas of robotics. For example, advanced control algorithms and predictive models developed for table tennis could be adapted for use in manufacturing, healthcare, and other industries where robots are required to perform complex tasks in dynamic environments.
The robot's performance against different levels of human players showcases the progress made in achieving human-level proficiency in a complex sport. Despite its current limitations, the research highlights the potential for further advancements in robotics, with broader implications for various industries.
Disclaimer: This article is intended to provide an overview of Google DeepMind's table tennis robot and its implications for robotics. The information presented is based on research and data available as of the publication date and is not an endorsement or promotion of any products or services.
Real-time information is available daily at https://stockregion.net